Keynote Speakers:
It is our pleasure to announce that two distinguished researchers give us keynote speeches.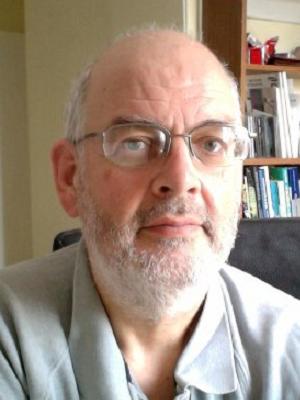
Dr. Inman Harvey
University of Sussex, England
Abstract: Evolutionary Robotics (ER) has now been used for many years for exploring the space of possible 'artificial brains', or 'brains + bodies' for robots and other AI or Artificial Life systems. For interesting behaviours these will be complex non-linear feedback systems, which are inherently difficult to design by conventional methods. Clearly natural Darwinian evolution has been successful for biological systems, hence it is thought to be useful for ER to copy this with Artificial Evolution.
The conventional picture is a Genetic Algorithm (GA) with a population (of genetically specified artificial systems, initially random), and successive generations where the 'fitter' members of each generation act as parents to pass on some of their characteristics, via their genes, to their offspring; over extended time, the population should evolve to become fitter. Fitness is here defined by the aims of the human controlling the artificial evolution, much as a farmer applies artificial selection to the breeding of cattle or rice. This conventional picture has vertical genetic transfer, from one generation to the next, and fitness is calculated for each individual. However very recent Metagenomic studies have painted a rather different picture of the microbial evolution that has comprised the first 2 billion years of evolution on this planet, together with the majority of evolution even today. For instance, a human being is a walking ecosystem that has coevolved with the microbial cells within; only 1% of the cells within the typical human-microbial ecosystem are actually human, and they are all coexisting in symbiosis.
So this new picture of evolution is one of horizontal gene transfer -- genes are swapped between microbes without the need for successive generations; and evaluation of fitness is at the symbiotic or community level, based on the viability of an ecosystem. Though one or other of these aspects have featured in some evolutionary computation before, here in the Binomic GA we incorporate both together for the first time. With rather simple code, we can evolve populations of component parts of a complex system, where fitness is evaluated at the system-level, and gene-transfer acts horizontally between different component parts.
This methodology is appropriate for complex systems where the behaviour of interest is at the community level arising from interactions between component parts: for instance individual agents within an agent swarm, or artificial neurons and connections within an Artificial Neural Network (ANN), or antibodies within an artificial immune system. We demonstrate its effectiveness with an ANN example.
* This talk is partially supported by the Japan Society of Mechanical Engineers Chugoku-Shikoku Branch.
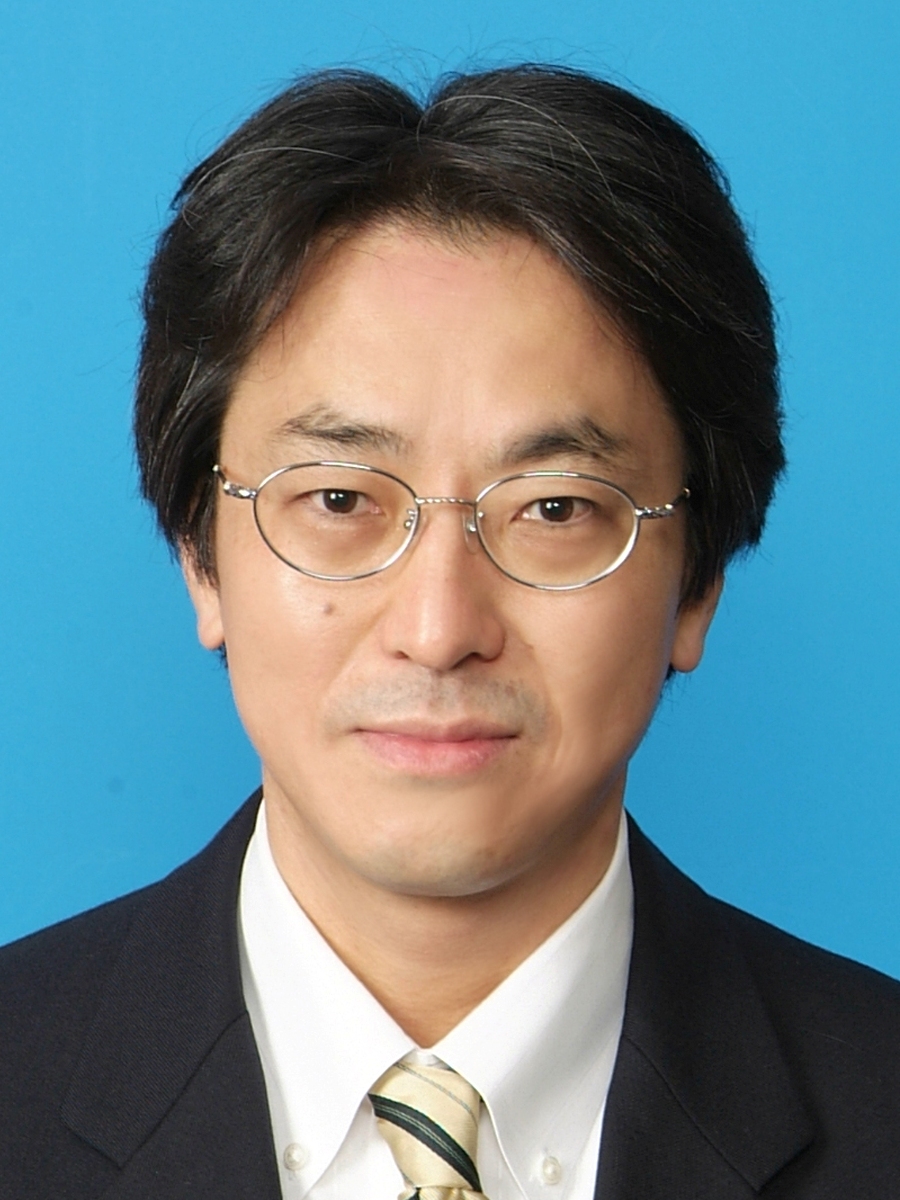
Dr. Hisao Ishibuchi
Osaka Prefecture University, Japan
Abstract: Evolutionary multi-objective optimization (EMO) has been one of the most active research areas in the field of evolutionary computation since the mid-1990s. Pareto dominance is used as the primary criterion for fitness evaluation in well-known and frequently-used EMO algorithms such as NSGA-II and SPEA. Whereas Pareto dominance-based EMO algorithms usually work very well on multi-objective problems with two or three objectives, they often show difficulties in the handling of many-objective problems with four or more objectives. This is because almost all individuals in each population become non-dominated with each other in very early generations when EMO algorithms are applied to many-objective problems. As a result, Pareto dominance-based fitness evaluation cannot drive multi-objective evolution toward the Pareto front. Thus good solutions close to the Pareto front are not likely to be obtained by Pareto dominance-based EMO algorithms for many-objective problems.
Recently the use of other fitness evaluation mechanisms has been actively studied for many-objective problems. One promising approach to many-objective optimization is the use of hypervolume as an indicator function for fitness evaluation. The fitness of each solution is measured by its contribution to the hypervolume of the current population. A multi-objective problem is handled as a single-objective hypervolume maximization problem in indicator-based EMO algorithms. Hypervolume maximization leads to good convergence toward the Pareto front and uniform distribution along the Pareto front even when multi-objective problems have many objectives. That is, good solution sets can be obtained by hypervolume maximization. Another promising approach to many-objective problems is the use of scalarizing functions. A number of scalarizing functions with different weight vectors are used to realize various search directions in the objective space. The main advantage of this approach is computational efficiency of scalarizing function calculation. Scalarizing functions are also used for local search in hybrid EMO algorithms. Hybridization with local search often significantly improves the search ability of EMO algorithms.
In this talk, first he will explains why Pareto dominance-based EMO algorithms do not work well on many-objective problems. Next he will give an overview of recent studies on EMO algorithms for many-objective optimization: Indicator-based and scalarizing function-based EMO algorithms. Then he will demonstrate advantages and disadvantages of those EMO algorithms such as heavy computation load of hypervolume calculation and high sensitivity to the choice of scalarizing functions. He will also explain how to combine local search with EMO algorithms. Finally he will suggest some future research directions.